Computational Linguistics Research in the Era of Artificial Intelligence-Christian Boitet
人工智能时代口译技术应用研究
王华树 | 国内首部聚焦口译技术应用和教学的著作
新书推荐
口笔译教育与评价国际论坛 二号公告
在厦门大学百年校庆之际,邀您齐聚厦门、共襄盛举
论坛推荐
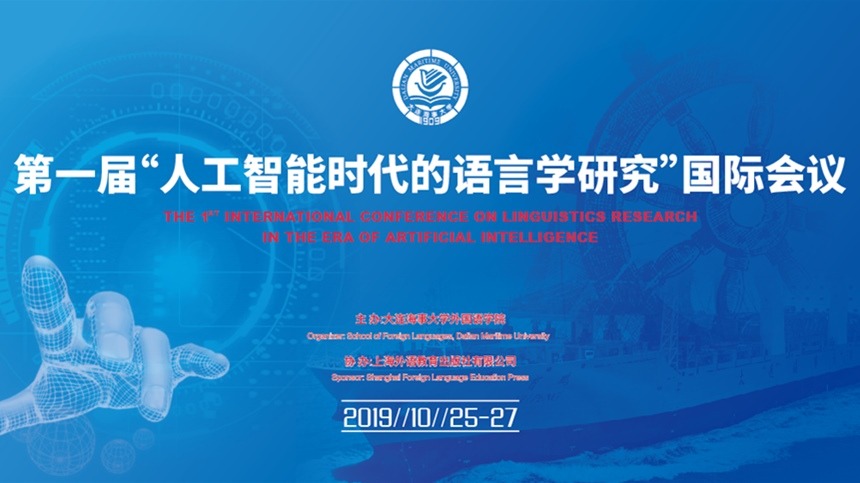
Computational Linguistics Research in the Era of Artificial Intelligence-Christian Boitet
第一届“人工智能时代的语言学研究”国际会议
观看大会全程直播
Christian Boitet
Dr. Christian Boitet is emeritus professor at Université Grenoble Alpes (UGA) and member of the LIG lab, after having been between 1977 and 2016 full professor of computer science at Université Joseph Fourier (UJF). He has taught programming, algorithmics, compiler construction, formal languages & automata, elementary logic, formal systems, and natural language processing. He is one of the authors of Ariane-G5, GETA’s generator of MT systems. He has presented communications in many national and international conferences, published in various journals and books, and edited a book dedicated to the presentation of Pr. Vauquois’ scientific work, as well as several international conference proceedings. He is a member of ICCL since 1988 and has been Programme co-Chair of COLING-1992 (Nantes), COLING-ACL’98 (Montréal), and COLING-2012 (Mumbai). He is and has been a regular reviewer for many journals and conferences, and has served in the programme committees of many congresses. His current interests include personal dialogue-based MT for monolingual authors (GETA’s LIDIA project, international UNL project), speech translation (aiming at the medical domain), machine helps to translators and interpreters, integration of speech processing inspired techniques in MT (hybrid approaches), multilingual lexical databases, and specialized languages and environments for lingware engineering and linguistic research (Ariane-Y project).
Computational Linguistics Research in the Era of AI
Abstract
This paper retraces the numerous interactions between Computational Linguistics (CL) and Artificial Intelligence (AI) since their earliest days, about 1950. The 1950-70 period saw the first modelling of natural languages (LN) through mathematical methods (formal ”static” grammars, dictionaries, transformational grammars) and the first Machine Translation projects, while AI centered on automatic (symbolic) theorem-proving and proposed the first Neural Net (NN), the Perceptron. A relatively large but high quality MT system had been demonstrated, PhD theses had produced the first automatic speech recognition (ASR) and synthesis (TTS) systems, and LUNAR, the first intelligent NL-based interface with a database, was operational.
The 1970-90 period saw the development of many MT operational systems (about 30 in Japan only), some with very large coverage, and a particular case, METEO, dedicated to weather bulletins, many expert systems were developed, based on knowledge bases fed by cogniticians, and CMU produced KBMT-89, the first prototype MT system using the ontology of the domain of its ”sublanguage”. During that period, empirical methods based on large sets of examples were developed, first for voice recognition, then for MT. And Harpy, based on Hidden Markov Models (HMMs) clearly beat knowledge-based systems.
The 1990-2010 period has been marked by R&D in speech translation on the CL side. International projects led by Japan (CSTAR) and Germany (VerbMobil) led to wearable systems, in particular Jibbigo (2010), working offline on mobile phones and tablets. On the written side, Example-Based MT (EBMT), an more specifically statistical MT (SMT), enjoyed an extraordinary development, leading to GoogleTranslate offering free large coverage MT between more than 100 languages. Knowledge-based systems like KANT (follower of KBMT-89) and MedSLT were mature, and AI had not only an immense success with Deep Blue beating Kasparov at chess in 1997, but also another one with Watson winning the Jeopardy! competition in 2011. Now, Information Retrieval (IR) could give way to intelligent question answering (Q/A), because structured information can be automatically extracted from NL documents without the need of cogniticians. A lesson of that period is the ”CAQ” metatheorem: for a given task like MT, or intelligent Q/A, coverage x automaticity x quality can never reach 100%. However, one can compromise on 1 factor and get the 2 others at (nearly) 100%. Another one, derived from research in EBMT, is that, in NL utterances, 96% of analogies of form are also analogies of meaning. That explains why form-based MT methods like EBMT and SMT (and later NMT) can work at all, and so well (on ”learnable” sublanguages).
We are in the middle of the 2010-2020 period. The most remarkable turn so far has been the use of very large multilayer (often convolutional) neural nets, rendered possible by the immense progress in hardware (GPUs as well as 512-core processors). But the ”human-specific” tasks of CL and AI, those (unlike face recognition or movement control) that differentiate humans from animals, are not handled in a satisfactory way, because the systems cannot explain their decisions, contrary to expert systems of the 80’s and the modern Q/A systems like Watson. Also, NN-based systems are very anti-ecological, gobbling up enormous amounts of computing power and computing space. That in turn leads to ethical problems: everything has to be processed ”on the Cloud”, and tasks or communities not having enough monetary (or linguistic) resources cannot be serviced. The final part of this paper outlines directions of research in CL that can lead to enabling multilingual access to information for speakers of the many languages that are currently under-resourced, although they are very active on the web.
《语言学研究2019》专题栏目
相关推荐
★★★★★ 5/5